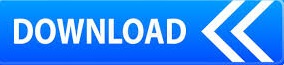
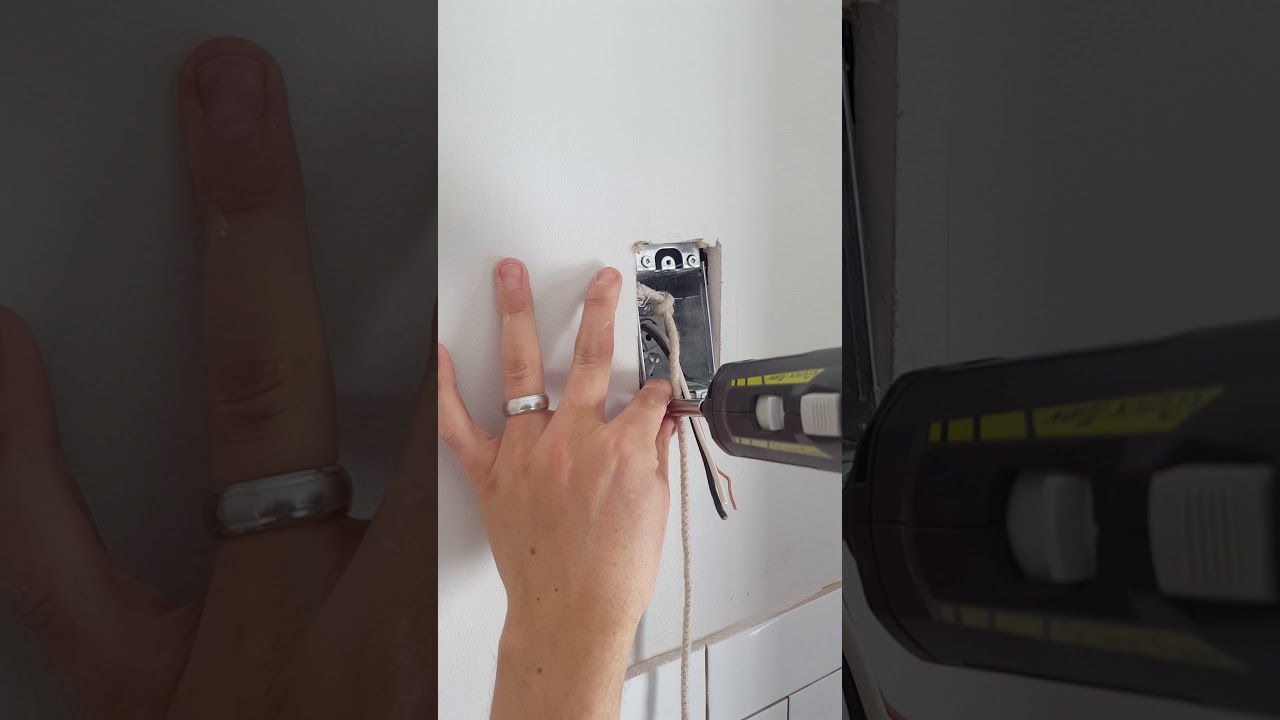
INBETWEENBOX TV
He thought TV should probe deeper, believing it could address big concerns: social injustice, bigotry, mortality. Always looking to skirt controversy, they preferred to work within the confines of formulaic Westerns and bland sitcoms. But corporate sponsors didn’t find his work appealing. His 1956 teleplay, Requiem for a Heavyweight, had garnered numerous awards, an Emmy among them. But the experience was too uncanny to forget.Īs a writer, Serling made his name toying with unsettling concepts, which made him a critical darling. He was a decade younger and, Serling joked, far better looking. “I kept staring and staring,” Serling recalled, “with this funny, ice-cold feeling that, if he turns around and it’s me, what do I do?” Eventually, the gentleman did turn around. As the award-winning TV writer tried to catch a glimpse of his double’s face, a strange thought hit him: What if, through some glitch in the universe, he was watching another version of himself? Across the room stood his doppelgänger: a man who looked to be his same height, sporting the same coat and carrying the exact same cowhide briefcase. As he waited to board his flight, he spotted something eerie. In the late 1950s, Rod Serling found himself sitting in a London airport tired and ready to go home. For Rod Serling, TV was the perfect landscape to battle bigotry and corporate censorship. Subscribe to our print edition here, and our iPad edition here.

To demonstrate the efficacy of our approach, we run a pilot study with users unfamiliar with robotic systems and analyze points of failure and future directions.This story originally appeared in print in the November 2014 issue of mental_floss magazine.
INBETWEENBOX SOFTWARE
We then develop an end-to-end system using open-source speech-to-text software and a Kinova Robotics MICO robotic arm. We develop our language model using data collected from Amazon Mechanical Turk to capture a comprehensive sample of terminology that people use to describe desired corrections. In this paper, we highlight four dimensions along which a user may wish to correct the behavior of his or her assistive manipulator. We then use the desired corrections to alter the behavior of the robotic manipulator by treating the modifications as constraints on the motion generation (planning) paradigm. Our natural language interface is based on the distributed correspondence graph, a probabilistic graphical model that assigns semantic meaning to user utterances in the context of the robot’s environment and current behavior. This work is applicable to users with and without disability. visual acuity and environmental knowledge). Allowing human operators to modify properties of how their robotic counterpart achieves a goal on-the-fly increases the utility of the system by incorporating the strengths of the human partner (e.g. This work is motivated by the desire to improve collaboration between humans and robots in a home environment. We propose a generalizable natural language interface that allows users to provide corrective instructions to an assistive robotic manipulator in real-time. Compared to Sequentially Constrained Monte Carlo (SCMC), which supports similar kinds of constraints, our SCHMC approach has faster convergence rates and lower parameter sensitivity. SCHMC significantly outperforms the Metropolis-Hastings algorithm (a standard Markov Chain Monte Carlo (MCMC) method) and the Hamiltonian Monte Carlo (HMC) method, in terms of both the accuracy of the sampling (for satisfying constraints) and the quality of approximation. We evaluate our approach for constrained sampling qualitatively and quantitatively with several classes of constraints. We are able to generate samples that satisfy arbitrarily complex, non-smooth and discontinuous constraints, which in turn allows us to support a wide range of qualitative information. Our approach, named Sequentially Constrained Hamiltonian Monte Carlo, integrates Hamiltonian dynamics into Sequentially Constrained Monte Carlo sampling. We introduce an algorithmic framework to model qualitative information as quantitative constraints on and between states. In human-robot collaborative tasks, incorporating qualitative information provided by humans can greatly enhance the robustness and efficacy of robot state estimation.
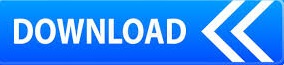